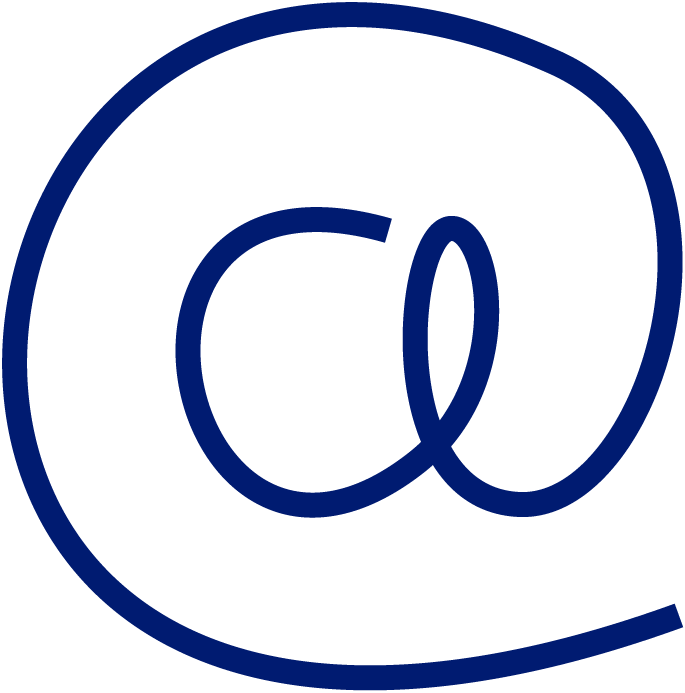
Artificial intelligence will be a powerful challengeto innovate in training. The goal is to make learners’ self-study moreeffective and equip trainers to guide and support them. Accordingly, we’d like you to examine three ways in which AI can be used to improve training and the learning environment.

To approach this issue, we’ve asked Marco Bertolini and Nora Yennek, both members of the Innovation by Cegos cluster, to provide an outside view on the matter and talk about their expectations.
Tree use cases for applying AI to training
In their book Artificial Intelligence in Education, Holmes, Bialik and Fadel put forward an initial taxonomy:
This taxonomy shows three fields in which AI can be applied to:
- A training activity or course, using data and analyses to continually adapt the user’s learning path;
- The learning environment: AI guides the learner to the right people and provides support for all of the non-training tasks (like a virtual concierge service);
- Optimising the learning process: AI becomes the trainer or course designer’s assistant. It provides specific recommendations for each learner or group and relieves the trainer or course designer of tasks with no added value in training terms.
In this blog post, we’ll be focusing on the first of these applications.
Read our first article on AI >>When artificial intelligence takes over training
AI applied to a learning path
In this use case, AI uses data and analyses to continually adapt the learning path to the learner. It will also change itself and improve with time, based on the data it captures. This approach can be described as “instructionist”. It involves learning content in a systematic, structured, explicit manner, progressing from the simple to the complex.
There are three levels for individualised learning systems. The first two are not based on AI, but the third is.

Differentiated learning
Within a given platform, learners can choose from several paths. They are generally organised into predefined categories. The learner chooses the most appropriate path for him or herself, without automatic guidance.
Personalised learning
There is a different learning path for each learner, suggested according to a rule-based method. For example, the learner can do a pre-assessment and, based on his or her answers, the system will suggest a learning path and specific content. This technology matches each learner’s profile to resources that are appropriate for him or her. Research shows that this type of personalisation improves learning outcomes. In short, the system assumes that each learner is completely unique and acts as a guide, mapping out the path to follow. Within Cegos and training courses such as the #UP collection, we use the Personalised Learning Path (PLP). This is a personalisation activity. Based on the learner’s opportunities to apply the learning content in the workplace, its guides the user to the most appropriate learning “sprints”.
Adaptive learning
Adaptive learning takes over when the personalised learning comes to an end. In this use case, AI uses data and analyses to continually adapt the learning path to the learner. It will also change and improve over time for each learner. This type of complex adaptability can be found today in specialised, research-driven programmes such as DreamBox (Math) and Carnegie Math. The programme adjusts the order and the material presented, depending on its analysis of the learner’s specific learning style and a deep, complex and iterative analysis of the student's understanding of a mathematical concept.
Various tools (Woonoz, Gutenberg Technology, Domoscio, Didask and Axonify) factor in some or all of these adaptive learning principles. However, the following two conditions are a minimum requirement for adaptive learning:
- Structure the content and break it down into separate learning objects, link them to specific knowledge or skills, and use metadata to describe them.
- Compile data about the learners, their learning style and how they assimilate concepts.
Let’s listen to Marco Bertolini on this subject:
In our next posts, we will discuss the other two fields of use: AI applied to the environment and AI applied to the learning process.
Article co-written by Fabienne Bouchut and François Debois.